Category: Agentic AI
10 minutes read
AI is About Transforming Human Ambition: Scaling What’s Possible
From Replacement to Reinvention: Unlocking Human Potential with AI
AI’s coming for our jobs— that’s the headline everyone is either terrified or tired of hearing. Though, this pervasive narrative isn’t entirely wrong— it’s catastrophically shortsighted. It reduces one of the most profound technological shifts in human history to a mere payroll issue.
For decades, we’ve been subtly trapped in a scarcity mindset – constrained by the finite bandwidth of human cognition, the limitations of accessible resources, and the inherent fragility of individual capacity. Every significant breakthrough, from penicillin to the moon landing, stands as a powerful testament to what human ambition could achieve despite these very constraints.
Now, AI changes the equation. Not by simply taking over tasks— but by fundamentally removing the constraints we’ve always taken for granted. And here, we’re not talking about faster spreadsheets or smarter assistants. We’re talking about unlocking a new operating system for human progress.
The printing press scaled knowledge; the steam engine scaled industry; the internet scaled connection.
AI? It scales ambition.
With AI, the lone innovator gains the potential for global collaboration, the impossible edges closer to the inevitable, and ambition itself is no longer solely bound by human bandwidth.
The myth is replacement; the truth is reinvention.
So, let’s stop asking what AI might take away—and start asking what it could help us build if limits were no longer part of the equation.
The Human Core of AI: Ambition, Not Automation
The spark that ignites every breakthrough is ambition. Ambition is an inherently human trait. AI, in this context, is not a competitor for this human characteristic, but rather a powerful collaborator. It provides the tools, the insights, and the capacity to translate our boldest visions into tangible realities.
Consider the relentless human ambition to conquer diseases and accelerate drug discovery. Here, AI’s sophisticated machine learning models can analyse vast genomic and proteomic datasets, identifying novel therapeutic targets and predicting drug efficacy with increasing precision. Algorithms can simulate molecular interactions and even design novel drug candidates, compressing the timelines of discovery from years to potentially months, bringing us closer to addressing previously intractable illnesses.
Similarly, the ambition to feed a growing global population sustainably finds a powerful ally in precision agriculture. Leveraging AI-powered analysis of satellite imagery, sensor data, and weather patterns, farmers can optimise resource allocation – water, fertilisers, pesticides – with unprecedented accuracy. Predictive models can anticipate potential yield impacts, enabling proactive interventions and maximising food production while minimising environmental footprint, paving the way for a more secure and sustainable food future.
Even the boundless human ambition to explore the cosmos is being profoundly amplified by AI in space exploration. Intelligent autonomous systems can navigate complex and hazardous environments on other planets, analyse geological data in real-time, and optimise spacecraft trajectories for interstellar missions. Further, machine learning algorithms can process the deluge of data from telescopes, identifying subtle anomalies and accelerating our understanding of the universe’s fundamental mysteries, extending our reach beyond Earth in ways previously deemed science fiction.
Across these critical domains, the unwavering engine of progress remains human ambition. As the transformative infrastructure, AI acts as a scalable force multiplier, exponentially accelerating our inherent drive and making the once impossible, inevitable.
Scaling Vision: From Local Insights to Global Action
The complex systems governing our world—climate, health, economics—are rich with data. Yet, insights often begin locally, sparked by specific observations, critical imperatives, or breakthroughs in human conceptualisation. AI then acts as the powerful engine to scale these local insights across contexts and borders, enabling meaningful global action.
Think of AI as a transformative network that not only amplifies these localised sparks of understanding but also intricately connects them across diverse ecosystems and industries, converting specific findings into globally impactful strategies. It accelerates the testing of locally developed solutions in varied environments and fosters systems thinking that integrates these ground-level understandings into a comprehensive global perspective.
Consider climate modelling. A local observation of an unusual glacial melt rate in the Himalayas, for instance, represents a specific, localised insight. When this data is fed into AI models that analyse global atmospheric patterns, ocean currents, and historical climate data from around the world, the localised insight gains global significance. AI can then predict the cascading effects of such melting on sea levels worldwide, inform international policy decisions on emissions targets, and guide the development of localised adaptation strategies based on global projections. The initial local insight becomes a crucial data point in a global understanding, driving worldwide action.
In pandemic forecasting, the initial identification of a cluster of novel pneumonia cases in a specific city represents a critical local insight. AI, when applied to global health data networks, travel patterns, and genomic sequencing of the pathogen, can scale this local information to a global level. By identifying potential transmission routes, predicting the speed and scale of spread across borders, and even forecasting regional healthcare capacity needs based on global trends, AI enables the development and deployment of worldwide intervention strategies, from vaccine distribution to lockdown measures. A localised medical observation becomes the trigger for global public health action.
Even in urban planning, a successful local initiative to optimise traffic flow using smart sensors in one city provides a valuable, context-specific insight. AI can analyse the data from this local implementation, compare it with traffic patterns and urban infrastructure data from cities around the world, and identify the underlying principles that can be generalised and applied globally. This allows urban planners worldwide to learn from local successes, adapt solutions to their own contexts, and ultimately create more efficient, sustainable, and livable cities based on insights gleaned from specific urban environments.
Thus, in every scenario, the initial insight, emerging from a particular context, is expanded by AI to inform and drive action on a global level. AI’s transformative power fuses local understanding with global data, creating solutions that address present needs while also illuminating pathways to navigate future complexities, fostering collective intelligence and action on an unprecedented scale.
Get in touch with us
Please provide your details and we’ll contact you
Subscribe to continue reading.
14 minutes read
Buy or Build your Life Sciences AI Sales Solutions?
About the Author
Richard Freeman holds an MEng in Computer Systems Engineering and a PhD in Artificial Intelligence from the University of Manchester. With over 22 years of experience in scalable big data, cloud computing, and advanced data science, he has worked with Fortune Global 500 companies and led high-impact initiatives—including Innovate UK-funded COVID-19 research and other high-risk, high-reward AI innovation projects.
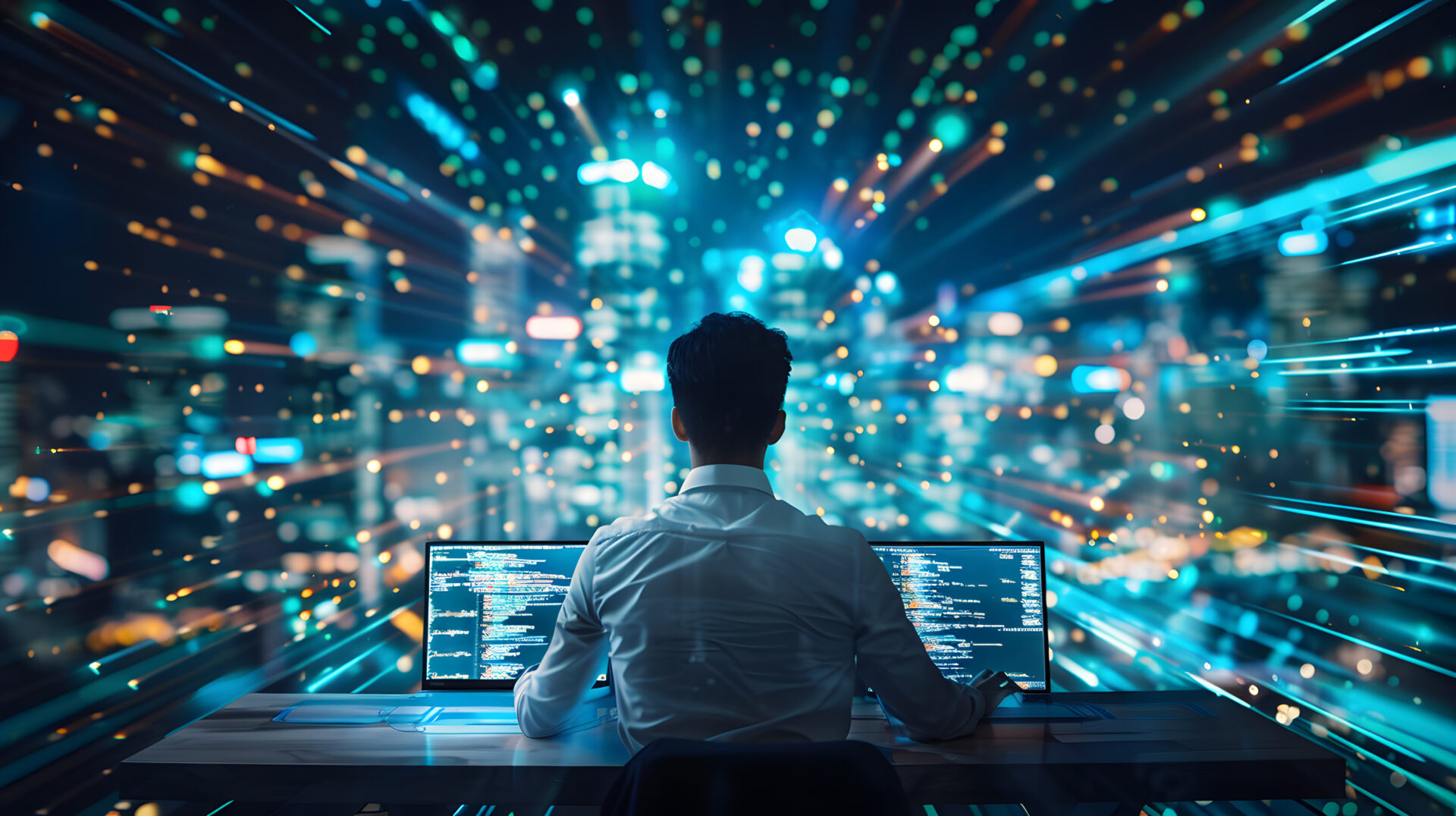
In today’s rapidly evolving Life Sciences industry, harnessing Generative AI to streamline and optimize sales opportunities has moved from futuristic vision to practical necessity to remain competitive – in the next 12 months 49% of healthcare companies are focusing on Generative AI1. Overall Generative AI in procurement is projected to grow to $2,260 million by 2032 with a CAGR) of 33%2.
Yet, despite widespread consensus on AI’s potential, organizations continually face the pivotal dilemma: should they buy an existing, tried-and-tested AI solution or build their own AI solution from the ground up?
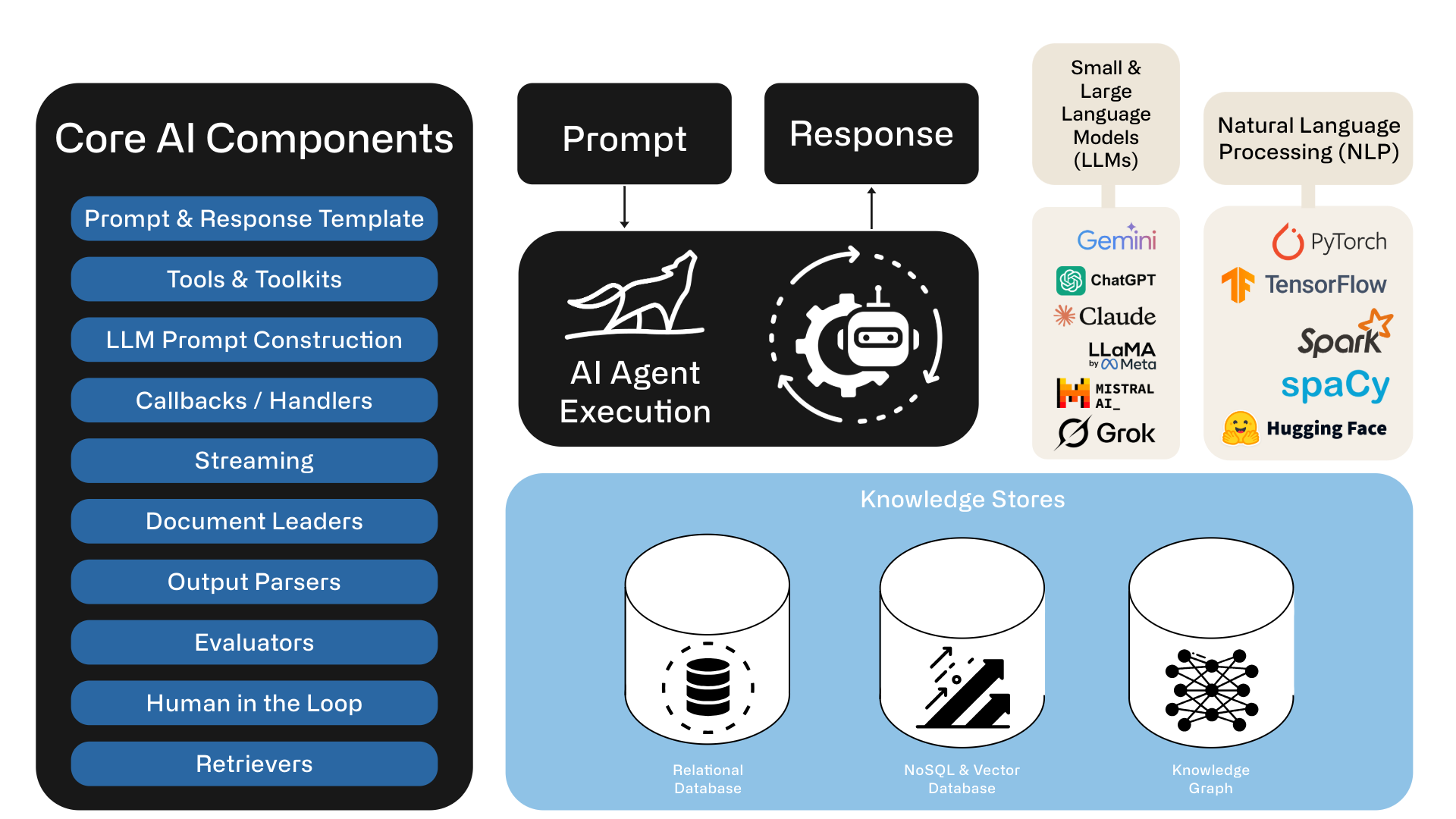
This decision is hardly trivial, and just because its AI doesn’t mean we can skip the best practices in architecture, software engineering, quality assurance, and monitoring. Implementing a robust AI-driven solution involves navigating through layers of complexities—ranging from agentic workflows, large language models (LLMs), knowledge graphs, natural language processing (NLP), data orchestration, and meticulous integration processes. Each comes with its own set of challenges, resources, time and costs.
Complexities of Building Your Own Production Agentic AI Workflow Solution
While building a fully customized AI solution can appear enticing—offering greater control, flexibility, and potential for tailored enhancements—it is notoriously complicated to scale beyond a pilot. Consider several critical factors that organizations often underestimate:
1. Architecture & Data Engineering
- Data Orchestration and Machine Learning Challenges: Building requires complex data and machine learning orchestration involving scraping, ingestion, extraction, normalization, matching, and maintaining data relevance. Life sciences data exist in highly heterogeneous records, country-specific data schemas, multi-lingual nuances, and more. Ensuring consistency and accuracy in data extraction and mapping is an immense and resource-intensive undertaking.
- Document & Knowledge Base Pre-processing: Ingesting data from various sources—such as SharePoint, Google Drive, or website can be far more complicated than it first appears. Different platforms may use unique authentication mechanisms, file structures, and access controls. Ensuring each source is parsed and standardized consistently takes careful planning and robust tooling. Metadata like version history or permissions often needs to be preserved for accuracy and compliance. If you skip thorough preprocessing, downstream processes (like text retrieval or indexing) may fail unexpectedly.
- Document Formats & PDF Issues: PDFs pose a unique challenge because they can contain anything from embedded fonts to scanned images, making extraction of clean text non-trivial. Other file types, like XLSX or DOCX, each come with their own peculiarities and parsing libraries. Even seemingly standard PDFs may have layout quirks that break text continuity or scramble logical paragraphs. Handling these inconsistencies often requires specialized conversion tools and a fair amount of manual intervention. Without a solid strategy to handle varied file formats, your RAG system risks being filled with fragmented or inaccurate text.
Without regularly updated, well organized and structured data, and the right context, most scalable AI systems will be slow, expensive, and not produce the expected outputs. It’s the garbage in garbage out problem - or in modern terms I call it feeding a Data Swamp (data/documents/media) to the AI Agent, and expecting it to figure it out, leading to creative fabricated responses !
2. Model Customization & Prompt Engineering
- Prompt Engineering and Domain Specificity: AI agents trained in life sciences require domain-specific embedding, fine-tuning, and rigorous prompt engineering for accuracy. An “off-the-shelf” generic LLM won’t deliver precision healthcare content or accurately understand nuanced domain criteria without extensive tuning and validation.
- Model Pipelines: A robust model pipeline integrates comprehensive version control to systematically track changes, updates, and iterations throughout the development process. This approach enables efficient rollbacks to stable versions when new iterations underperform or introduce issues, ensuring minimal downtime and consistent performance.
If your AI model is truly in a production environment, and making you money, saving time or helping customers, it can also go rogue, wild or wrong and do the opposite - how to quickly can you revert it to its best working state?
3. Quality Assurance (QA) & Monitoring
- Stochastic Nature and Extraction Variability: LLMs exhibit inherent stochasticity; identical prompts may yield variable outputs. This variability makes consistency difficult to achieve, especially in regulated industries like Life Sciences, where uniformity and predictability are paramount. DIY solutions thus necessitate intricate preprocessing and post-processing validation steps, including explicit frameworks and guardrails for continuous quality management.
- Accuracy Problems in Production: During testing, your model might work on a limited set of well-curated data and predictable queries, creating a false sense of security. However, in real-world usage, users can ask unanticipated questions or supply ambiguous input that the system hasn’t been trained or tuned for. This can result in incorrect or incomplete answers at critical times, damaging trust and adoption. Monitoring and continual validation become essential once the system is live. You may need to introduce feedback loops and additional fine-tuning based on actual user interactions to maintain high accuracy.
- Hallucinations: Large Language Models can generate coherent yet entirely fabricated information, known as “hallucinations.” These outputs often appear authoritative, making them dangerous for decision-making if users don’t double-check the facts. Minimizing hallucinations requires careful prompt engineering, model configuration, or the addition of retrieval-based evidence to back up answers. Even then, you need robust post-processing checks to filter out spurious content. Ultimately, effective oversight—human or algorithmic—is essential for mitigating the risk of misleading answers.
- Response Quality Assurance: Evaluating the quality of AI-generated text isn’t as straightforward as running unit tests or verifying numeric outputs. You need to assess factors like relevance, factual correctness, clarity, and style—many of which are inherently subjective. Automated metrics (e.g., BLEU, ROUGE) often don’t reflect real-world user satisfaction or correctness. Consequently, many organizations introduce human-in-the-loop review to catch errors and gather feedback. However, scaling this human QA process can be labor-intensive and expensive, especially for large deployments.
- Tip: create your own curated dataset for automated testing and measuring
4. Software Engineering & Integration
- Integration with Existing Systems: Your RAG or Agentic AI solutions rarely operate in isolation; they often need to integrate with databases, CRMs, ticketing systems, or other enterprise applications. Each system has its own data schema, APIs, authentication processes, and performance constraints that must be respected. A mismatch or lack of synchronization in these integrations can lead to erroneous data, delays, or even security vulnerabilities. Moreover, once users rely on automated answers drawn from external systems, they expect data to be up-to-date. Orchestration tools and well-documented APIs become vital to avoid integration chaos.
- Software Engineering: By embracing a holistic framework that integrates DevOps, DataOps, MLOps, and AIOps, and by adhering to best software engineering practices, design patterns, and principles, organizations can streamline data pipelines, automate deployments, and achieve scalable, secure, and efficient systems across the entire development, deployment, and operational lifecycle.
5. Compliance, Security & Governance
- Compliance & Audit Requirements: Regulatory standards like GDPR, HIPAA, ISO, or SOC-2 mandate strict controls over how data is accessed, processed, and stored. When dealing with text ingestion and generation, you must track the flow of potentially sensitive information and maintain audit logs. Failure to do so can result in non-compliance penalties, legal troubles, and reputational damage. Additionally, meeting these standards often requires specialized security reviews, documentation, and secure cloud deployments. Balancing agility and innovation with strict regulatory requirements can significantly slow development cycles.
- Security & Data Leakages: Ingesting private, proprietary, or personal data into AI systems raises red flags for security-minded stakeholders. An improperly configured system might inadvertently expose confidential information in generated responses, store that data in logs accessible to unauthorized personnel or share the data in public GenAI APIs. Use of private models, encryption at rest, encryption in transit, robust access controls, and careful logging policies become table stakes. You also have to consider potential vulnerabilities in any third-party integrations or APIs. If you don’t build with security in mind from the outset, you risk severe breaches that can undermine the entire project.
Tip: Embed security ground up and involve the right stakeholders early
Leveraging Existing Agentic Workflow Solutions
In contrast, buying and implementing an existing specialized life sciences, medtech, pharmaceutical, or biotech agentic AI solution mitigates these complexities significantly. Specialized solutions come ready-equipped with agentic workflows, prebuilt reflection patterns, domain-specific embeddings, and optimized integration modules. Pre-tested and penetration-certified, they ensure security and compliance out-of-the-box, with proven consistency in performance and minimized variability.
Immediate Usability and Integration
Prebuilt solutions are typically designed to seamlessly integrate into existing ERP, CRM, and CPQ workflows, significantly reducing implementation friction. Users benefit from predefined schemas, consistent data extraction, and multi-language support, allowing fast deployment and immediate usability without additional manual overhead.
Scalability and Cost Efficiency
At scale, ready-to-use solutions reduce the total cost of ownership through optimized infrastructure, centralized updates, and vendor-provided support. Organizations can immediately benefit from consistent, validated, and standardized analytics without the resource drain of continuous maintenance and internal troubleshooting.
Real-world AI Use Cases from working with the top ten medtech firms
Consider scenarios such as capturing highly detailed award criteria from diverse documentation, accurately extracting nuanced healthcare data, performing country-specific analytics across varying languages, and normalizing the data values and quantities. At a small scale you can resolve some of this with prompt engineering and processing the documents manually. In an enterprise grade solution you may have thousands of opportunities with multi-language scanned documents that need to be processed daily. Such tasks involve highly sophisticated AI orchestrations—tasks that are painstakingly complicated to accurately replicate internally but efficiently addressed by specialized vendor solutions that have already done extensive groundwork and have production-grade core AI components.
Additionally, vendors providing specialized Life Sciences AI solutions have performed extensive penetration testing, satisfying rigorous security standards required for sensitive data handling and regulatory compliance. Attempting such certification independently is both resource-intensive and risk-prone.
Buy vs. Build: Strategic Considerations
To build or to buy? Answering this pivotal question revolves around several key strategic considerations:
- Time-to-Value: DIY solutions are slow to develop, while buying a prebuilt agentic workflow solution provides quicker ROI and value realization.
- Complexity and Risk: Building involves substantial complexity, internal expertise, and continuous risk mitigation, while buying mitigates these significantly.
- Domain Specialization: Existing solutions are already extensively tuned and validated against the nuances of pharma/biotech/medech-specific criteria and regulations.
- Scalability and Maintenance: At large scale, prebuilt solutions offer significantly greater operational efficiency, lowering overhead, and ongoing maintenance burdens.
Conclusion: Thoughtful Decisions, Strategic Impact
While the allure of bespoke AI solutions is understandable, the significant technical, domain-specific, regulatory, and operational complexities associated with building your own solution in Life Sciences cannot be overstated. Specialized AI agentic workflow platforms offer solid integration, robust security and compliance, proven domain specificity, and optimal scalability.
Organizations keen on strategic advantage and swift ROI realization will likely find that choosing to buy an existing specialized Life Sciences AI solution significantly reduces complexity and cost, ensuring robust and immediate operational enhancement. Building your own AI solution, though attractive in theory, presents substantial and nuanced practical hurdles that few organizations are fully prepared to overcome efficiently.
In navigating AI in Life Sciences, recognizing when to leverage existing specialist AI partners such as Vamstar (I’m the CTO and co-founder) can be your greatest strategic advantage, rapid deployment and return on investment.
Other Articles
13 minutes read
What Every MedTech CEO Should Know About Unlocking the Potential of AI in Commercialisation
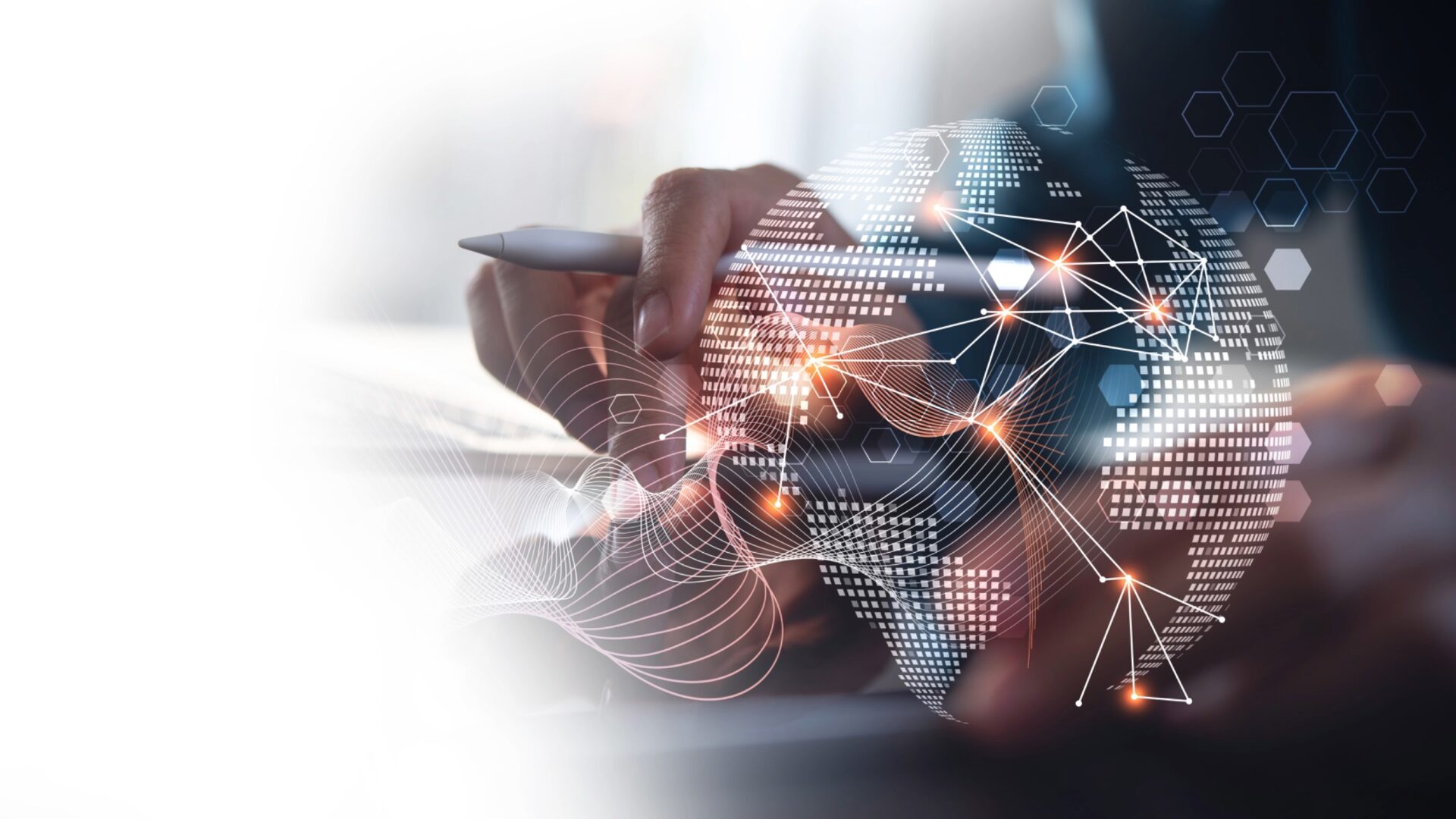
Artificial Intelligence (AI) is revolutionising the MedTech industry, offering significant opportunities to enhance commercialisation strategies. As a MedTech CEO, understanding how to effectively integrate AI into your business operations is crucial for maintaining a competitive edge.
Here are key considerations to unlock AI’s potential in commercialisation:

1. Leverage AI for Market Entry and Expansion
AI-driven data analysis enables precise market entry strategies by identifying emerging trends, regulatory requirements, and customer needs. Platforms like Vamstar’s Polaris facilitate this by seamlessly integrating data across healthcare markets, enabling MedTech companies to map, track, and analyse policy landscapes and evidence bases. This alignment with market demands and sustainability frameworks, such as Value-Based Procurement (VBP) processes, ensures companies can address both immediate and long-term goals.
Empowering Market Access through Agentic AI takes this a step further. Agentic AI is an advanced, autonomous solution designed to support market access teams at local, regional, and global levels. By autonomously gathering, mapping, tracking, and analysing essential evidence bases and policies, it provides dynamic insights and actionable intelligence. This enables teams to make informed decisions and drive value-based procurement strategies with unparalleled precision.
Key Features of Agentic AI:
- Autonomous Evidence Mapping: Using Vamstar’s Polaris, clinical evidence is independently collected, classified, and synthesised, forming a strong foundation for strategic decision-making.
- Policy Intelligence: Vamstar’s Polaris continuously monitors and evaluates regulatory changes and policy shifts, helping teams stay aligned with the evolving market landscape.
- Global Market Surveillance: Polaris aggregates data from over 86,000 buyers across more than 100 countries, delivering a comprehensive view of global market dynamics.
Benefits:
- Optimised Market Access: Delivers real-time insights into evidence and regulatory landscapes, streamlining market entry and expansion.
- Increased Operational Efficiency: Automates data collection and analysis, reducing manual effort while improving accuracy.
- Strategic Decision-Making: Provides data-driven insights that align with organisational goals and market forces, driving sustainable growth.
Applications:
- Value Mapping: Maps value and pricing dynamics autonomously across payer landscapes, integrating data from diverse markets and sources.
- Sustainability Monitoring: Tracks and evaluates industry-wide sustainability initiatives, ensuring seamless integration with ecosystem partners.
- Value-Based Procurement (VBP): Facilitates VBP adoption by providing clarity on contract outcomes, improving operational efficiency, and aligning cost-of-care criteria with purchasing decisions.
Agentic AI revolutionises traditional market access strategies by autonomously driving insights and aligning organisations with the evolving demands of global markets. Opinion leaders highlight that delaying AI adoption or underestimating its value can leave companies behind in a fast-evolving market.
2. Streamline Operations and Decision-Making
Implementing AI can optimise various operational aspects, from supply chain management to sales forecasting. AI algorithms predict demand, manage inventory, and streamline logistics, resulting in increased efficiency and reduced costs. Industry leaders suggest that the integration of AI in these areas allows companies to remain agile and responsive, avoiding common pitfalls like resource mismanagement and operational silos.
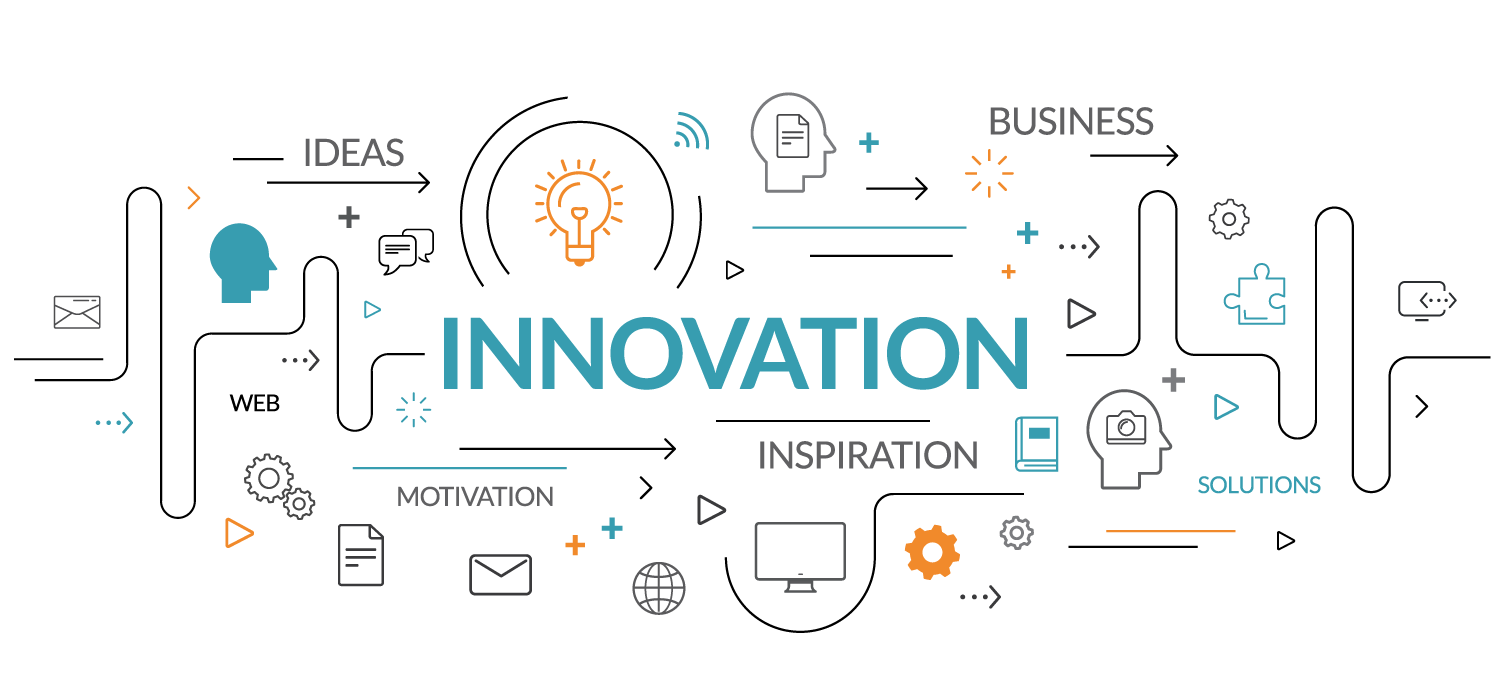
3. Foster Innovation in Product Development
AI facilitates accelerated innovation by analysing complex datasets, such as patient outcomes, clinical trial data, and real-world evidence, to identify new product opportunities and improvements. These datasets provide insights into unmet needs, treatment efficacy, and market dynamics, enabling companies to innovate with precision and confidence.
Generative AI, for instance, can assist in designing novel medical devices or enhancing existing ones by enabling advanced simulation, rapid prototyping, and predictive modelling. These capabilities allow teams to explore numerous design iterations quickly, optimise device performance, and predict real-world outcomes, ensuring your product offerings remain at the forefront of technological advancement.
According to Deloitte’s survey, 42% of MedTech executives report significant benefits from AI in product development, including cost reductions and new revenue streams. Leaders also emphasise that AI-driven collaboration across R&D, production, and marketing teams accelerates innovation, ensuring adaptability in a fast-evolving market.
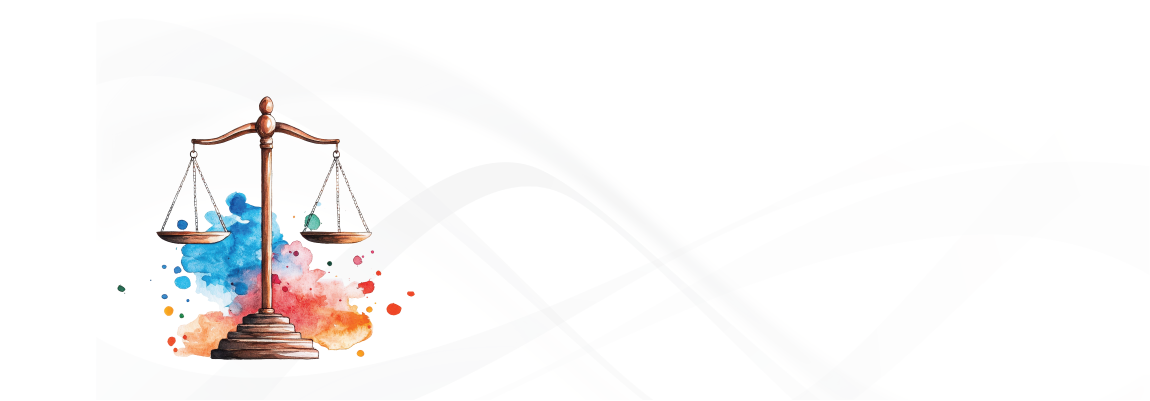
5. Invest in AI Talent and Culture
Building a team with AI expertise is vital for successful implementation. To operationalise this:
- Conduct a Skills Gap Analysis: Assess your organisation’s current AI-related skills and identify areas that need development.
- Hire Strategically: Recruit professionals with expertise in AI technologies, data science, and industry-specific applications. Consider hiring an AI-focused Chief Innovation Officer or forming an advisory board with AI experts.
- Invest in Training Programs: Provide ongoing training for existing employees to build AI literacy and upskill in areas like data management and AI ethics.
- Foster Cross-Functional Teams: Encourage collaboration between technical, operational, and strategic teams to ensure AI initiatives align with business goals.
- Promote a Culture of Innovation: Implement programs that reward innovative uses of AI, such as hackathons or internal AI competitions.
- Leverage External Partnerships: Collaborate with universities, research institutions, or AI-focused organisations to stay updated on the latest technologies and methodologies.
- Evaluate Progress and Impact: Regularly measure the success of AI initiatives in achieving business goals using KPIs.
6. Avoid Common Pitfalls in AI Implementation
Avoiding common missteps in AI implementation is critical for success. Overestimating AI capabilities or underestimating the importance of data quality can lead to inefficiencies and missed opportunities. Leaders caution against waiting for perfect conditions to adopt AI, advocating instead for phased implementation strategies that adapt to evolving business needs and technological advancements.
Vertical-specific AIs, such as Vamstar’s Polaris, tailored to address specialised challenges, offer transformative potential. These solutions not only improve operational efficiency but also deliver actionable insights that align closely with market demands, ensuring a competitive edge in dynamic environments. By focusing on incremental deployment and aligning AI solutions with specific business objectives, organisations can maximise the value of their AI investments while minimising risks.
7. Incorporate AI in Sustainability Efforts
AI tools can optimise resource usage, reduce waste, and streamline operations in line with sustainability goals. This is particularly relevant for Value-Based Procurement (VBP) processes, where AI helps align commercial activities with environmental and social objectives. Advanced AI capabilities, including AI-driven ESG reporting and resource tracking, enable MedTech companies to meet sustainability goals while enhancing operational efficiency.
8. Optimise Pricing Strategies with AI
Pricing is a core focus for MedTech and pharmaceutical companies, and leveraging AI in pricing processes offers transformative potential. AI-powered tools analyse vast datasets, including market trends, competitor pricing, and historical sales data, to develop dynamic pricing models that adapt to real-time conditions.
Key Benefits:
- Improved Accuracy: Advanced machine learning algorithms identify patterns and predict market behaviours, ensuring precision in pricing strategies.
- Increased Efficiency: Automation accelerates decision-making, enabling rapid adjustments to market changes with optimised pricing.
- Enhanced Profitability: AI balances competitiveness with profitability, ensuring that pricing strategies maximise margins while remaining attractive to customers.
Applications:
- Dynamic Pricing Models: Adjust prices in real time based on market demand and competitive positioning.
- Revenue Optimisation: Use predictive analytics to identify optimal price points that maximise revenue and market share.
- Contract Pricing Support: Streamline and harmonise pricing across contracts, ensuring consistency and compliance.
Real-World Impact: Implementing AI-powered pricing solutions has shown measurable success. For instance, companies have reported significant improvements in bidding success rates and margin optimisation by adopting AI-driven tools. These capabilities not only improve profitability but also foster strategic decision-making that aligns with organisational goals.
By integrating AI into pricing strategies, MedTech and pharmaceutical companies can navigate market complexities more effectively, optimise revenue streams, and maintain a competitive edge in an increasingly dynamic industry landscape.
9. Emphasise Real-World Evidence Integration
AI platforms synthesise and analyse real-world evidence (RWE) from diverse sources such as electronic health records, patient registries, and wearables. These platforms not only validate product efficacy but also enhance the credibility of regulatory submissions by integrating comprehensive, high-quality data. Additionally, they support value-based discussions with stakeholders by offering actionable insights into treatment outcomes and patient needs. By transforming raw data into structured intelligence, they enable MedTech companies to anticipate market shifts, align with payer priorities, and strengthen their competitive positioning in an evidence-driven landscape.
10. Strengthen Post-Market Surveillance
AI-driven monitoring enables the detection of adverse events, flags potential risks, and ensures strict adherence to post-market regulatory requirements. By leveraging continuous AI-powered surveillance, MedTech companies can proactively identify emerging issues, reduce response times, and maintain high standards of product safety and compliance. This capability not only safeguards patients but also reinforces trust with regulatory bodies and stakeholders, strengthening the organisation’s reputation in the industry.
Conclusion
By focusing on these critical areas, MedTech CEOs can harness the full transformative power of AI to revolutionise commercialisation, enhance operational efficiency, and foster innovation. AI is no longer just a tool; it is a strategic enabler that seamlessly integrates across all functions, driving sustainable growth and equipping organisations to proactively navigate industry shifts. With a forward-thinking and comprehensive approach, AI empowers MedTech companies to maintain their competitive edge, achieve resilience, and create a lasting impact in a rapidly evolving and highly competitive healthcare landscape.
Transform Your Organisations Growth
Our expertise and innovative solutions, are designed to empower MedTech companies to unlock new opportunities, optimise commercial operations, and stay ahead of the curve.
Get in touch with us
Please provide your details and we’ll contact you.
Other Articles
7 minutes read
Global Leadership in Agentic AI in Lifesciences
Agentic AI is reshaping the global life sciences sector, particularly within Pharma, MedTech, and BioTech, by delivering advanced commercial capabilities. Across regions, the potential to lead in strategic guidance, market access insights, and pricing strategies is driving the adoption of autonomous AI systems.
While North America and Europe remain pivotal players, the Asia-Pacific (APAC) and Middle East regions are emerging as strategic hubs for commercial agentic AI innovations.
This article explores the global landscape, with a focus on APAC and the Middle East, highlighting their roles in driving transformation within life sciences.
APAC: A Hub for Innovation and Scalability
The Asia-Pacific region is rapidly becoming a global leader in leveraging agentic AI for life sciences commercialisation. Countries like China, Japan, and South Korea are at the forefront, utilising AI to enhance market access, optimise pricing strategies, and provide actionable insights for market expansion.
Key Developments:
- Strategic Market Access in China: China’s extensive investments in AI-driven health technology have enabled companies to develop predictive models for market entry and reimbursement strategies. Agentic AI tools analyse regional payer requirements, ensuring products align with local policies and accelerate approvals.
- Dynamic Pricing in Japan: Japanese firms are leveraging AI to develop dynamic pricing strategies that respond to market demand fluctuations and regulatory constraints. This ensures optimal profitability while maintaining compliance.
- AI-Enhanced Customer Engagement in South Korea: AI systems in South Korea are driving tailored communication strategies for healthcare providers, improving the positioning of MedTech solutions and fostering stronger stakeholder relationships.
APAC’s focus on scalability and data availability positions it as a leader in deploying agentic AI solutions that address diverse market needs.
The Middle East: Emerging Strategic AI Leadership
The Middle East, particularly the United Arab Emirates (UAE) and Saudi Arabia, is positioning itself as a key player in commercial agentic AI for life sciences. With substantial investments in AI innovation and infrastructure, the region is setting benchmarks for integrating AI into market access and pricing strategies.
Key Developments:
- Market Access Optimisation in the UAE: The UAE’s National AI Strategy 2031 emphasises the use of agentic AI to navigate complex reimbursement landscapes. Companies are leveraging AI-driven insights to predict payer behaviours and tailor access strategies.
- Pricing Innovation in Saudi Arabia: Saudi initiatives are focused on developing AI models that analyse regional economic conditions and healthcare expenditure patterns. These insights are used to create adaptive pricing frameworks that balance affordability with profitability.
- Talent and Infrastructure Development: Institutions like the Mohammed bin Zayed University of Artificial Intelligence (MBZUAI) are cultivating local expertise, ensuring the region’s capability to innovate and implement AI solutions for commercial applications.
North America: A Benchmark for Strategic AI Deployment
North America, led by the United States, continues to set the standard for commercial agentic AI applications. Pharma and MedTech companies utilise AI to enhance decision-making, improve market responsiveness, and maintain competitiveness.
Key Developments:
- Dynamic Pricing and Market Intelligence: Companies like IQVIA are leveraging AI to deliver dynamic pricing insights that incorporate competitor strategies, market demand, and payer policies.
- Strategic Partnerships: Collaborations between AI leaders (e.g., OpenAI) and life sciences firms are fostering innovation in market access tools and predictive pricing models.
Europe: Ethical and Sustainable AI Leadership
Europe’s emphasis on ethical AI development makes it a unique player in the global landscape. The EU’s AI Act ensures transparency and accountability, aligning agentic AI applications with sustainable commercial goals.
Key Developments:
- AI-Driven Reimbursement Models: European companies are using AI to predict reimbursement success by analysing real-world evidence and health economic data.
- Regulatory Navigation: Agentic AI assists firms in aligning with diverse regulatory requirements across EU member states, ensuring seamless market entry.
Africa and Emerging Markets: Niche Applications
Emerging markets in Africa are utilising agentic AI for niche applications, particularly in market access and pricing optimisation for underserved regions.
Key Developments:
Localised Market Access Solutions: AI systems in Kenya and South Africa analyse regional data to support life sciences companies in tailoring affordable pricing strategies for low-income populations.
Commercial Applications Driving Life Sciences Transformation
Across these regions, agentic AI is delivering unprecedented value in key commercial functions:
- Market Access Insights: Predicting reimbursement success and tailoring strategies to regional payer landscapes.
- Dynamic Pricing Models: Responding to market demand and regulatory shifts in real time.
- Strategic Decision-Making: Providing actionable intelligence for market expansion and resource allocation.
- Customer Engagement: Personalising interactions with healthcare providers and payers to enhance commercial outcomes.
- Operational Efficiency: Streamlining pricing governance and contract management.
Conclusion
Agentic AI is transforming the commercial dynamics of the life sciences industry. While North America and Europe remain benchmarks, APAC and the Middle East are emerging as critical players, driving innovation through unique regional strengths. By integrating agentic AI into market access, pricing, and strategic decision-making, these regions are shaping the future of Pharma, MedTech, and BioTech industries, ensuring global competitiveness and sustainable growth.
Explore Our AI Solutions
Vamstar hosts a variety of AI capabilities to enable your organisation to grow effectively.
Get in touch with us
Please provide your details and we’ll contact you