9 minutes read
The Evolution of AI in Medical Devices: Regulatory Challenges and Future Directions
Artificial intelligence (AI) and machine learning (ML) are reshaping the MedTech landscape, driving advancements in diagnostic precision, personalised treatment, and operational efficiency. At the Advamed MedTech conference, industry leaders and regulatory representatives from Canada and the U.S. explored the integration of AI into medical devices, spotlighting its transformative potential and the regulatory frameworks needed to harness it responsibly.
As of August 2024, nearly 1,000 AI/ML-enabled medical devices have received regulatory approval. This milestone underscores AI’s growing prominence but also highlights a pressing need to address the unique challenges posed by adaptive, self-learning technologies. Ensuring safety, efficacy, and ethical use in a rapidly evolving field demands bold, forward-thinking regulatory strategies.
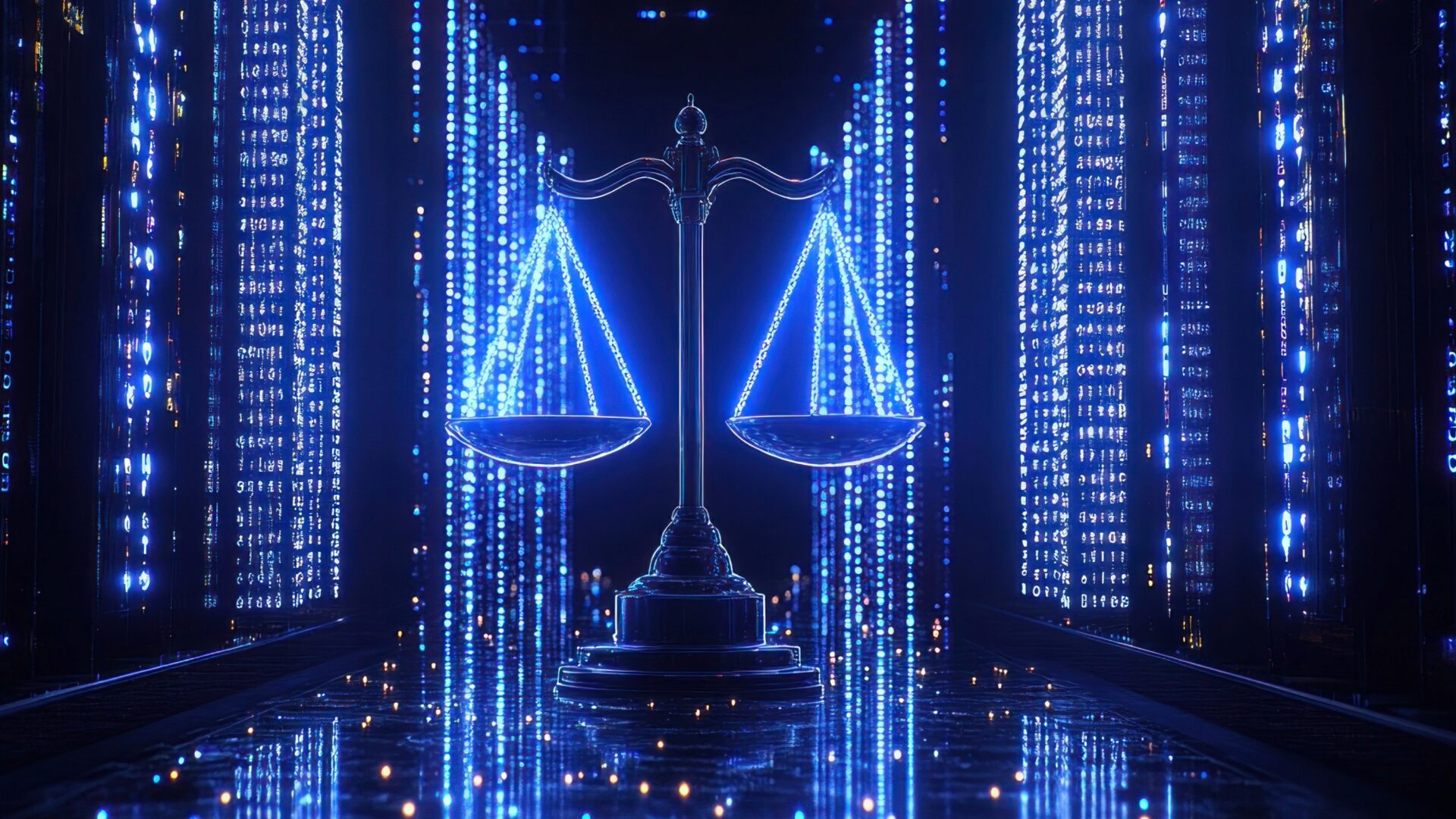
The AI Revolution in Medical Devices
AI in medical devices dates back to the 1990s, with early applications in imaging that relied on locked, static algorithms. Today, adaptive AI models dominate, capable of evolving with new data and contexts. These dynamic capabilities unlock revolutionary potential—personalised care, faster diagnostics, and smarter healthcare systems—but also present regulatory complexities far beyond traditional device oversight.
To keep pace, the MedTech industry and regulators are rethinking frameworks that historically relied on static product definitions and pre-market approvals. The result is a growing emphasis on continuous monitoring, adaptive oversight, and cross-sector collaboration.
Leading Regulatory Efforts: Health Canada and FDA
Regulatory agencies are stepping up to meet the demands of AI-driven innovation:
- Health Canada’s Digital Health Division: Established in 2018, this team oversees high-risk AI medical devices, focusing on cybersecurity, software, and adaptive learning technologies. It is instrumental in setting Canada-specific performance benchmarks and lifecycle guidelines.
- FDA’s Digital Health Center of Excellence: Pioneering frameworks for AI/ML in healthcare, the FDA is evolving its regulatory philosophy to balance rapid innovation with uncompromising safety standards.
The Key Regulatory Challenges
- Performance Degradation
Adaptive AI models can “drift,” where performance declines over time as data environments change. Regulators are pushing for real-time monitoring frameworks to ensure safety and efficacy throughout a device’s lifecycle. - Transparency and Explainability
The complexity of AI models often creates a “black box” effect, making it difficult to understand how decisions are made. Regulators are driving initiatives to improve transparency—enabling stakeholders to trust AI systems without necessarily understanding their full complexity. - Post-Market Surveillance
AI’s ability to evolve post-deployment necessitates a shift in regulatory focus from pre-market evaluations to robust, ongoing performance monitoring. Agencies are piloting adaptive models for oversight to align with AI’s continuous development. - Evolving Regulatory Frameworks
Decades-old regulatory structures, designed for static devices, are ill-suited for AI’s dynamic nature. Agencies like the FDA and Health Canada are redefining what constitutes a medical device, establishing iterative approval processes, and exploring pathways for rapid updates. - Cross-Site Deployment Challenges
AI models trained in one environment may underperform in different settings. Regulators and manufacturers are collaborating on protocols for local adaptation and validation to ensure consistent performance across diverse clinical contexts. - Healthcare Workforce Pressures
AI is increasingly viewed as a solution to alleviate workforce shortages. Regulators are balancing the need for AI deployment speed with safeguards to ensure human oversight, ethical integration, and clinician training. - Data Silos
Fragmented healthcare datasets hinder AI model development. Regulatory agencies are working to break down silos through frameworks for federated learning, synthetic data generation, and secure, privacy-compliant data sharing.
Regulatory Advances and Innovations
Health Canada’s Strategic Initiatives
- Machine Learning Guidance: A forthcoming guidance document will set clear lifecycle standards for AI devices, focusing on adaptability and continuous improvement.
- License Conditions: Tailored conditions ensure devices meet performance benchmarks even after deployment.
- Collaborative Stakeholder Engagement: Partnerships across industry, academia, and healthcare inform Canada’s regulatory priorities.
- Scientific Advisory Committee: This expert-led group guides digital health strategies, emphasizing AI policy development.
- Predetermined Change Control Plans (PCP): These plans enable manufacturers to implement pre-approved updates, reducing regulatory bottlenecks.
FDA’s Forward-Thinking Framework
- Standards Development: Aiming for universal benchmarks, the FDA is crafting evaluation standards tailored to AI technologies.
- Risk Management Protocols: Comprehensive quality assurance systems monitor AI lifecycle risks and mitigate evolving threats.
- Nuanced Evaluation: By distinguishing between predictive and generative AI, the FDA tailors regulatory reviews to the specific characteristics of each technology.
- Proactive Education: Outreach efforts are equipping stakeholders—from legislators to clinicians—with the knowledge to navigate AI’s integration.
Global Harmonisation
Initiatives such as the International Medical Device Regulators Forum (IMDRF) and joint publications from Health Canada, the FDA, and the UK’s MHRA aim to standardise global AI regulatory approaches, fostering consistency across jurisdictions.
Emerging Concepts Shaping Regulation
- Transparency vs. Explainability: Regulators are prioritising transparent communication of AI capabilities while recognising that full explainability may not be feasible for all models.
- Human-AI Collaboration: Frameworks increasingly consider AI as an augmentation tool, emphasising its integration into clinician workflows rather than standalone decision-making.
- Lifecycle Management: Adaptive oversight ensures safety across the entire AI device lifecycle, from development to decommissioning.
- Bias Mitigation: AI must demonstrate equity in outcomes across diverse patient populations, driving stricter subgroup analyses and bias identification protocols.
- Benefit-Risk Evolution: Regulatory assessments now weigh long-term, systemic benefits, even in cases where traditional statistical backing may be limited.
Future Directions
- Defining Medical Devices in an AI Era
AI blurs the line between software and hardware, prompting regulators to rethink device classifications and approval categories. - Accelerating Regulatory Timelines
Rolling submissions and phased approvals are being explored to keep pace with AI advancements without compromising safety. - Focus on Underserved Populations
Targeted incentives are encouraging AI development for paediatric care and underrepresented demographics, addressing equity gaps in healthcare. - Standardising Transparency
Frameworks like model cards and data cards will become essential tools for communicating AI limitations and capabilities. - System-Wide AI Integration
AI’s potential extends beyond devices to addressing workforce shortages and cost challenges. Regulators are exploring frameworks that consider AI’s broader systemic impact.
Conclusion
The integration of AI into medical devices represents one of the most significant paradigm shifts in modern healthcare. While the benefits—improved accuracy, personalised care, and operational efficiency—are immense, these advancements also demand regulatory frameworks that are as dynamic and adaptable as the technologies themselves.
By embracing lifecycle management, fostering transparency, and prioritising cross-stakeholder collaboration, regulators can strike the delicate balance between fostering innovation and safeguarding patient safety. As AI reshapes the MedTech landscape, its responsible integration will define the next era of healthcare, delivering transformative solutions to global challenges. The future is here, and it’s adaptive, intelligent, and deeply collaborative.
Discover Our Solutions
Discover how we can empower your business with Agentic AI solutions and drive growth.
Get in touch with us
Please provide your details and we’ll contact you