Category: Uncategorized
7 minutes read
Lisbon: Shaping the Intelligent Future of Healthcare
A Hub for MedTech Innovation and Vision
The MedTech Forum, Europe’s largest health and medical technology conference, returns to Lisbon from May 13-15, 2025. This isn’t just an event; it’s a dynamic platform where the cutting edge of MedTech meets the future of healthcare. Building on the success of the 2024 Vienna forum, the 2025 edition promises to be a crucial gathering for industry leaders, innovators, and policymakers.
Key Learnings from Vienna 2024: Building Momentum
From AI Potential to Practice
The 2024 Forum in Vienna made it clear: AI’s role in diagnostics, personalised medicine, and operations is no longer theoretical. But successful adoption hinges on more than technology—it demands rigorous validation, integration into clinical workflows, and ethical oversight. Key themes included data privacy, algorithm transparency, and cross-disciplinary collaboration between developers and healthcare providers.
Regulatory Clarity: Still a Moving Target
The evolving regulatory environment, particularly around AI and digital health, remains a pressing challenge. The EU MDR’s ongoing impact was a dominant topic, along with calls for more agile regulatory pathways that support innovation while safeguarding patient safety.
Patient-Centricity Isn’t Optional—It’s Imperative
Patient-centric innovation must go beyond buzzwords. The Forum highlighted the need for solutions that address real-world patient needs and challenges—while ensuring equitable access to emerging technologies.
Collaboration as a Strategic Necessity
No single stakeholder can drive transformation alone. Forums like this emphasise the power of coordinated ecosystems—uniting MedTech companies, clinicians, regulators, payers, and researchers under shared goals.
Interoperability & Data Security: The Bedrock of Digital Health
Data that can’t be shared—or isn’t secure—undermines progress. Seamless data exchange and strong cybersecurity measures are foundational for connected care systems.
Lisbon 2025: What to Expect
AI Integration, at Scale
Sessions at Lisbon 2025 will present concrete examples of AI embedded within clinical and administrative workflows. Expect demonstrations of AI-driven diagnostic imaging that enhances early cancer detection accuracy, potentially reducing diagnostic timelines and accelerating treatment decisions. The agenda also includes deep dives into AI’s role in remote patient monitoring, particularly its impact on chronic disease management and reducing hospital readmissions.
The AIxHealthcare track will feature domain experts addressing challenges in scaling AI across varied healthcare settings, with greater focus on ensuring interoperability, equity, and clinical trust. Discussions will also touch on AI-enabled procurement and how intelligent automation is transforming market access strategies.
Reimagining Regulatory Models
Striking the right balance between innovation and safety is a central theme in Lisbon. Panels will explore how current frameworks, such as the EU MDR, IVDR, and the proposed AI Act can evolve to better serve patients and innovators alike. Topics will include the use of real-world evidence to accelerate regulatory approval for AI-powered devices, the potential for adaptive regulatory pathways, and the need for greater international harmonisation.
Expect critical insight from stakeholders including regulatory agencies, policymakers, and MedTech leaders on how to create agile frameworks that keep pace with rapid technological advancement without compromising patient safety.
Designing with (and for) the Patient
Lisbon 2025 will go beyond the rhetoric of patient-centricity. Workshops will explore how to embed patient involvement throughout the product development lifecycle—from co-design sessions to interpreting patient feedback and usability testing.
There will be sessions on creating intuitive user interfaces tailored to diverse patient populations, and examples of how virtual and augmented reality are being deployed to enhance patient education and engagement.
Enabling a Truly Collaborative Ecosystem
Through networking events and partnership-driven sessions, Lisbon 2025 aims to accelerate collaboration across the value chain—from R&D to commercialisation and care delivery. Structured networking events will connect early-stage startups with MedTech giants, while panels will explore how public-private partnerships are advancing precision medicine and digital health initiatives.
Other sessions will highlight interdisciplinary cooperation among clinicians, data scientists, and engineers—showcasing how these synergies drive meaningful innovation in real-world care settings.
Securing and Unlocking Data
In a data-driven healthcare landscape, security and accessibility must go hand in hand. Sessions will explore how to protect sensitive health data in an age of rising cyber threats, with best practices for implementing robust digital defences.
Expect expert perspectives on federated learning, GDPR-compliant AI training, and privacy-preserving technologies that enable research and innovation without compromising confidentiality.
The ‘CEO #Nofilter Session’
A standout feature of Lisbon 2025 is the CEO #nofilter session—an open and candid dialogue with leading industry executives, including Annette Bruls (Edwards Lifesciences), Gavin Wood (J&J MedTech), Urmi Prasad Richardson (Thermo Fisher Scientific), and Roland Goette (BD). This session, scheduled for May 15th from 8:30 to 9:20 AM, promises to delve beyond polished presentations, offering unfiltered insights into the challenges, opportunities, and strategic decisions shaping the future of healthcare. Expect discussions on navigating regulatory volatility, scaling patient-centric innovation, building resilient teams, and shaping future-ready business models.
Spotlight: Vamstar’s Role in the Future of AI in MedTech
As a platinum sponsor, Vamstar is set to be instrumental in shaping the dialogue around the future of AI in MedTech at the forum. Our commitment to driving innovation and efficiency in the healthcare sector will be highlighted in our speaking session: “AI Unleashed: Strengthening MedTech Competitiveness in a New Industrial Age.“
This session will delve into concrete strategies for MedTech leaders to leverage AI for tangible competitive advantages in Europe’s dynamic healthcare landscape. Attendees can expect to gain valuable insights into navigating regulatory complexities, optimising routes to market, accelerating sales cycles, and mitigating cybersecurity risks—all while maintaining a crucial patient-centric approach.
Vamstar’s expertise promises to offer a practical roadmap for unlocking AI’s transformative power in the MedTech industry. Our presence will underscore how intelligent automation is already delivering measurable business outcomes across the MedTech value chain.
Will MedTech Lead, or Follow?
The MedTech Forum 2025 isn’t just a checkpoint—it’s a turning point. The decisions, alliances, and innovations shaped in Lisbon will define how the sector meets the next era of healthcare challenges. The question for every stakeholder is clear: Will you lead with AI, or simply adopt it? Will you drive change, or merely respond?
The future of healthcare is intelligent—and MedTech must decide what role it wants to play.
10 minutes read
Bridging the Atlantic: Communicating Europe’s AI-Driven Healthcare Innovations to US Stakeholders
What does it take for a breakthrough AI diagnostic tool developed in France or Sweden to gain traction in Boston or Chicago?
The answer lies not just in its algorithms, but in its ability to strategically communicate within the US healthcare system.
Europe is fast becoming a powerhouse for AI-driven healthcare, with innovations emerging from a mix of startups, university spin-outs, and publicly funded research initiatives. As these technologies mature, many face a familiar hurdle: navigating the commercial and regulatory complexities of the United States—still the world’s largest and most competitive healthcare market. From FDA classifications to fragmented reimbursement structures, the transatlantic journey for European MedTech is marked by both opportunity and nuance.
To succeed, European innovators must go beyond clinical excellence. They need to adapt their strategies to the unique demands of the US landscape—aligning with the expectations of regulators, payers, and procurement leaders through targeted communication, robust evidence generation, and AI-driven market intelligence.
Divergent Landscapes: Regulation and Market Expectations
Europe’s approach to AI in healthcare is shaped by a rigorous, ethics-first framework. The EU AI Act, provisionally adopted in 2024, classifies AI systems by risk and imposes strict obligations on high-risk applications—healthcare among them. These rules work in tandem with the EU Medical Device Regulation (MDR), which governs the clinical evaluation, safety, and performance of medical devices and diagnostic technologies. Together, they form a comprehensive but complex compliance environment that prioritises patient safety and data transparency.
In contrast, the US regulatory environment, anchored by the Food and Drug Administration (FDA), favours a more adaptive, iterative model. The FDA has introduced several regulatory pathways for digital health and AI/ML-based medical devices, including:
- 510(k) premarket notification, which allows manufacturers to demonstrate that their device is substantially equivalent to an already approved product. This is often a faster and less costly route to market.
- De Novo classification, designed for novel devices with no direct predicate, enabling innovators to bring first-of-their-kind technologies to market with a reasonable assurance of safety and effectiveness.
- The Breakthrough Devices Program, which offers expedited review for technologies addressing life-threatening or irreversibly debilitating conditions.
Rather than a single overarching regulation like the EU AI Act, the FDA operates through evolving guidance documents and pilot initiatives, such as the AI/ML Software as a Medical Device (SaMD) Action Plan, which supports innovation while focusing on algorithm transparency, real-world performance monitoring, and adaptive learning systems.
While the FDA’s flexible approach can enable faster market access, its lack of harmonisation presents both opportunity and risk. Therefore, European innovators must reframe their compliance narratives to meet US expectations and present a compelling case for both safety and scalability.
AI-Powered Insights to Guide Market Strategy
The complexity of entering the US healthcare market can be mitigated with AI-driven intelligence that maps demand trends, procurement behaviours, and reimbursement models. With the right tools, innovators can uncover patterns in stakeholder adoption, financial viability, and technology uptake timelines to identify:
- US healthcare systems adopting AI diagnostics or robotic surgical systems.
- Reimbursement pathways established by Medicare and private insurers for AI-powered solutions through new CPT (Current Procedural Terminology) codes—the billing codes used by clinicians and hospitals to report medical procedures and services.
- Opportunities where data from routine clinical practice influences formulary listings and hospital technology assessments.
Unlike Europe’s nationally standardised DRG-based reimbursement systems, the US market is highly fragmented—comprising public and private payers, each with distinct coverage policies. Even clinically approved solutions must undergo rigorous economic value assessments before they gain traction.
Get in touch with us
Please provide your details and we’ll contact you
Subscribe to continue reading.
14 minutes read
Buy or Build your Life Sciences AI Sales Solutions?
About the Author
Richard Freeman holds an MEng in Computer Systems Engineering and a PhD in Artificial Intelligence from the University of Manchester. With over 22 years of experience in scalable big data, cloud computing, and advanced data science, he has worked with Fortune Global 500 companies and led high-impact initiatives—including Innovate UK-funded COVID-19 research and other high-risk, high-reward AI innovation projects.
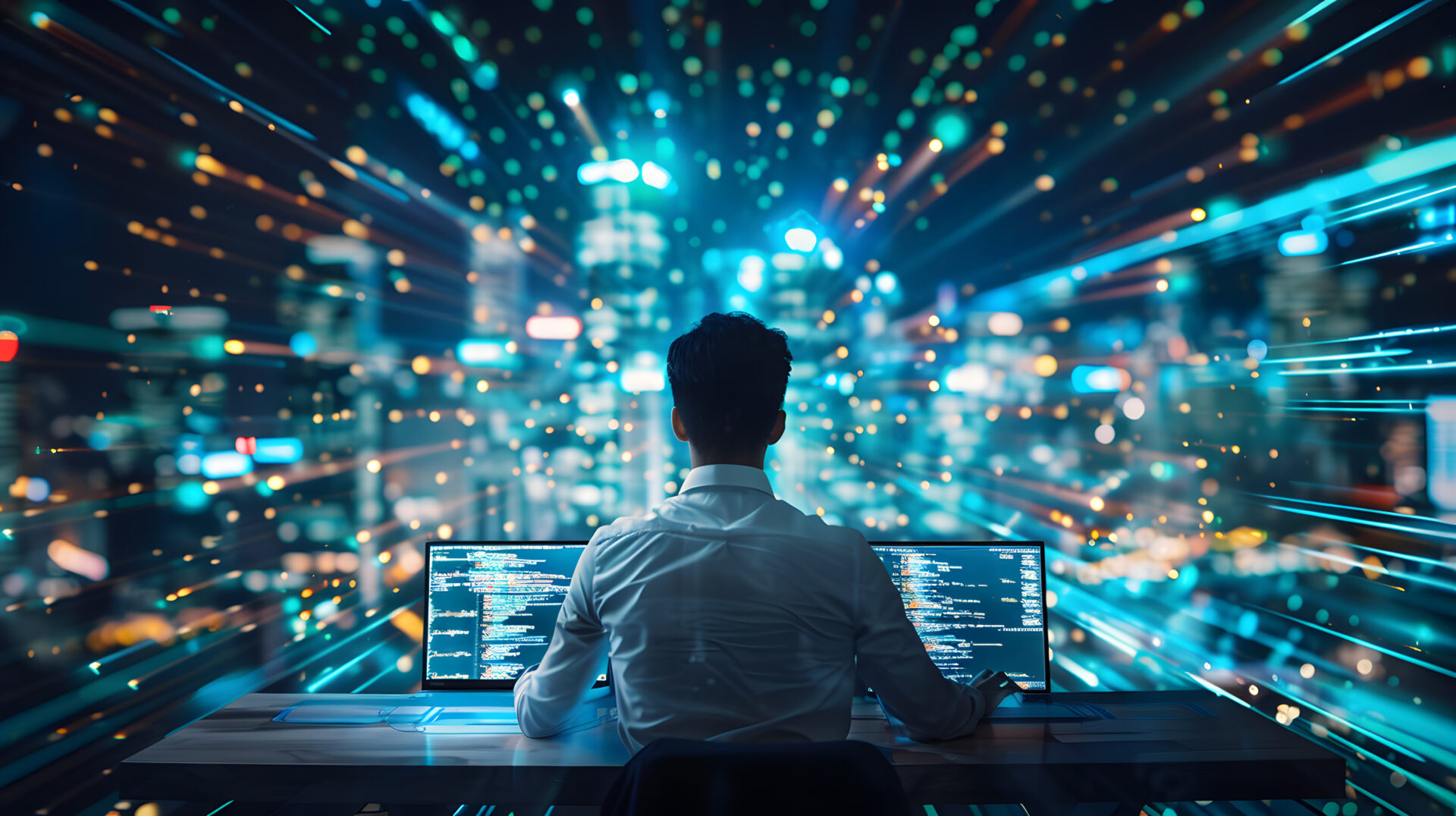
In today’s rapidly evolving Life Sciences industry, harnessing Generative AI to streamline and optimize sales opportunities has moved from futuristic vision to practical necessity to remain competitive – in the next 12 months 49% of healthcare companies are focusing on Generative AI1. Overall Generative AI in procurement is projected to grow to $2,260 million by 2032 with a CAGR) of 33%2.
Yet, despite widespread consensus on AI’s potential, organizations continually face the pivotal dilemma: should they buy an existing, tried-and-tested AI solution or build their own AI solution from the ground up?
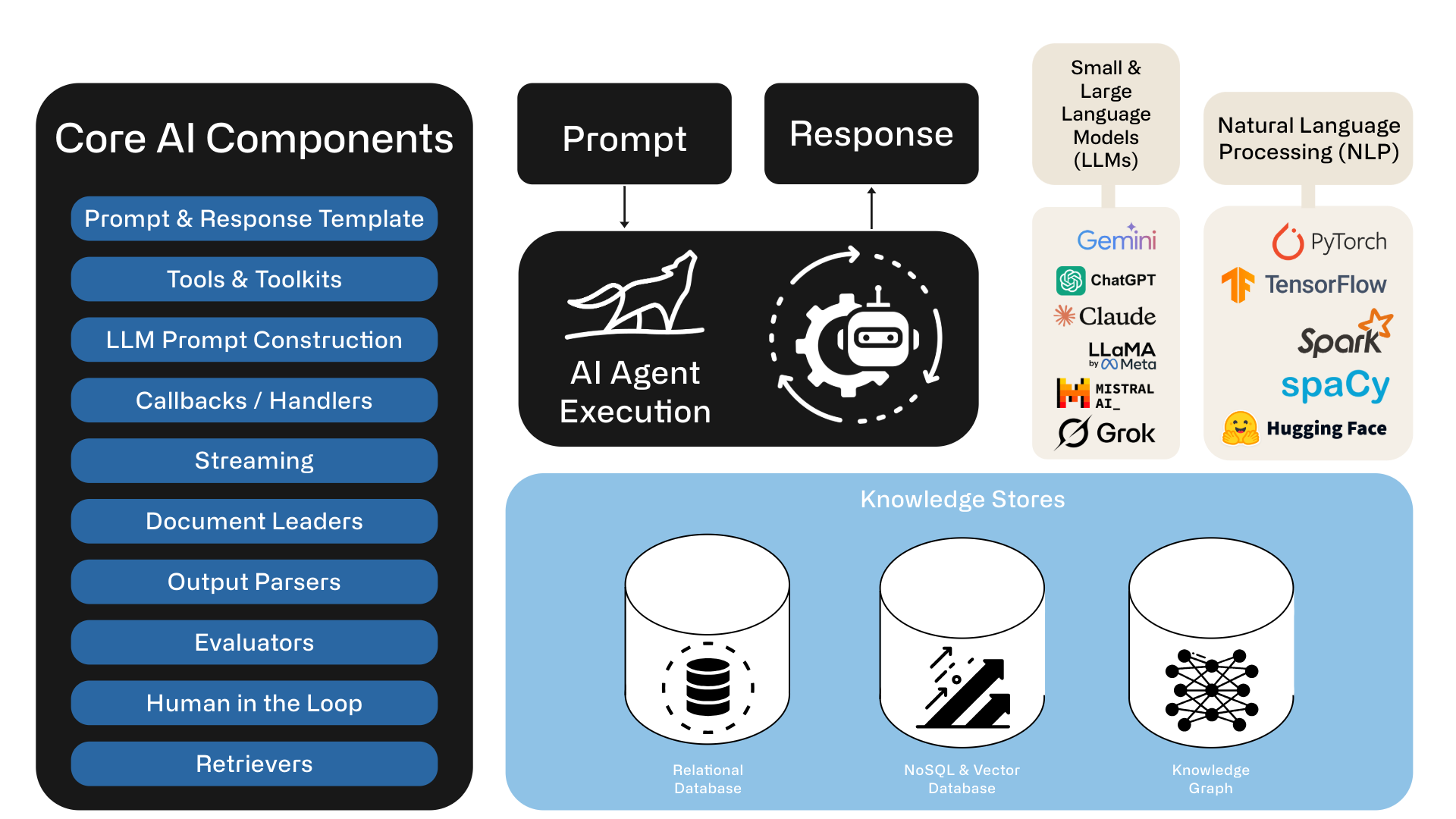
This decision is hardly trivial, and just because its AI doesn’t mean we can skip the best practices in architecture, software engineering, quality assurance, and monitoring. Implementing a robust AI-driven solution involves navigating through layers of complexities—ranging from agentic workflows, large language models (LLMs), knowledge graphs, natural language processing (NLP), data orchestration, and meticulous integration processes. Each comes with its own set of challenges, resources, time and costs.
Complexities of Building Your Own Production Agentic AI Workflow Solution
While building a fully customized AI solution can appear enticing—offering greater control, flexibility, and potential for tailored enhancements—it is notoriously complicated to scale beyond a pilot. Consider several critical factors that organizations often underestimate:
1. Architecture & Data Engineering
- Data Orchestration and Machine Learning Challenges: Building requires complex data and machine learning orchestration involving scraping, ingestion, extraction, normalization, matching, and maintaining data relevance. Life sciences data exist in highly heterogeneous records, country-specific data schemas, multi-lingual nuances, and more. Ensuring consistency and accuracy in data extraction and mapping is an immense and resource-intensive undertaking.
- Document & Knowledge Base Pre-processing: Ingesting data from various sources—such as SharePoint, Google Drive, or website can be far more complicated than it first appears. Different platforms may use unique authentication mechanisms, file structures, and access controls. Ensuring each source is parsed and standardized consistently takes careful planning and robust tooling. Metadata like version history or permissions often needs to be preserved for accuracy and compliance. If you skip thorough preprocessing, downstream processes (like text retrieval or indexing) may fail unexpectedly.
- Document Formats & PDF Issues: PDFs pose a unique challenge because they can contain anything from embedded fonts to scanned images, making extraction of clean text non-trivial. Other file types, like XLSX or DOCX, each come with their own peculiarities and parsing libraries. Even seemingly standard PDFs may have layout quirks that break text continuity or scramble logical paragraphs. Handling these inconsistencies often requires specialized conversion tools and a fair amount of manual intervention. Without a solid strategy to handle varied file formats, your RAG system risks being filled with fragmented or inaccurate text.
Without regularly updated, well organized and structured data, and the right context, most scalable AI systems will be slow, expensive, and not produce the expected outputs. It’s the garbage in garbage out problem - or in modern terms I call it feeding a Data Swamp (data/documents/media) to the AI Agent, and expecting it to figure it out, leading to creative fabricated responses !
2. Model Customization & Prompt Engineering
- Prompt Engineering and Domain Specificity: AI agents trained in life sciences require domain-specific embedding, fine-tuning, and rigorous prompt engineering for accuracy. An “off-the-shelf” generic LLM won’t deliver precision healthcare content or accurately understand nuanced domain criteria without extensive tuning and validation.
- Model Pipelines: A robust model pipeline integrates comprehensive version control to systematically track changes, updates, and iterations throughout the development process. This approach enables efficient rollbacks to stable versions when new iterations underperform or introduce issues, ensuring minimal downtime and consistent performance.
If your AI model is truly in a production environment, and making you money, saving time or helping customers, it can also go rogue, wild or wrong and do the opposite - how to quickly can you revert it to its best working state?
3. Quality Assurance (QA) & Monitoring
- Stochastic Nature and Extraction Variability: LLMs exhibit inherent stochasticity; identical prompts may yield variable outputs. This variability makes consistency difficult to achieve, especially in regulated industries like Life Sciences, where uniformity and predictability are paramount. DIY solutions thus necessitate intricate preprocessing and post-processing validation steps, including explicit frameworks and guardrails for continuous quality management.
- Accuracy Problems in Production: During testing, your model might work on a limited set of well-curated data and predictable queries, creating a false sense of security. However, in real-world usage, users can ask unanticipated questions or supply ambiguous input that the system hasn’t been trained or tuned for. This can result in incorrect or incomplete answers at critical times, damaging trust and adoption. Monitoring and continual validation become essential once the system is live. You may need to introduce feedback loops and additional fine-tuning based on actual user interactions to maintain high accuracy.
- Hallucinations: Large Language Models can generate coherent yet entirely fabricated information, known as “hallucinations.” These outputs often appear authoritative, making them dangerous for decision-making if users don’t double-check the facts. Minimizing hallucinations requires careful prompt engineering, model configuration, or the addition of retrieval-based evidence to back up answers. Even then, you need robust post-processing checks to filter out spurious content. Ultimately, effective oversight—human or algorithmic—is essential for mitigating the risk of misleading answers.
- Response Quality Assurance: Evaluating the quality of AI-generated text isn’t as straightforward as running unit tests or verifying numeric outputs. You need to assess factors like relevance, factual correctness, clarity, and style—many of which are inherently subjective. Automated metrics (e.g., BLEU, ROUGE) often don’t reflect real-world user satisfaction or correctness. Consequently, many organizations introduce human-in-the-loop review to catch errors and gather feedback. However, scaling this human QA process can be labor-intensive and expensive, especially for large deployments.
- Tip: create your own curated dataset for automated testing and measuring
4. Software Engineering & Integration
- Integration with Existing Systems: Your RAG or Agentic AI solutions rarely operate in isolation; they often need to integrate with databases, CRMs, ticketing systems, or other enterprise applications. Each system has its own data schema, APIs, authentication processes, and performance constraints that must be respected. A mismatch or lack of synchronization in these integrations can lead to erroneous data, delays, or even security vulnerabilities. Moreover, once users rely on automated answers drawn from external systems, they expect data to be up-to-date. Orchestration tools and well-documented APIs become vital to avoid integration chaos.
- Software Engineering: By embracing a holistic framework that integrates DevOps, DataOps, MLOps, and AIOps, and by adhering to best software engineering practices, design patterns, and principles, organizations can streamline data pipelines, automate deployments, and achieve scalable, secure, and efficient systems across the entire development, deployment, and operational lifecycle.
5. Compliance, Security & Governance
- Compliance & Audit Requirements: Regulatory standards like GDPR, HIPAA, ISO, or SOC-2 mandate strict controls over how data is accessed, processed, and stored. When dealing with text ingestion and generation, you must track the flow of potentially sensitive information and maintain audit logs. Failure to do so can result in non-compliance penalties, legal troubles, and reputational damage. Additionally, meeting these standards often requires specialized security reviews, documentation, and secure cloud deployments. Balancing agility and innovation with strict regulatory requirements can significantly slow development cycles.
- Security & Data Leakages: Ingesting private, proprietary, or personal data into AI systems raises red flags for security-minded stakeholders. An improperly configured system might inadvertently expose confidential information in generated responses, store that data in logs accessible to unauthorized personnel or share the data in public GenAI APIs. Use of private models, encryption at rest, encryption in transit, robust access controls, and careful logging policies become table stakes. You also have to consider potential vulnerabilities in any third-party integrations or APIs. If you don’t build with security in mind from the outset, you risk severe breaches that can undermine the entire project.
Tip: Embed security ground up and involve the right stakeholders early
Leveraging Existing Agentic Workflow Solutions
In contrast, buying and implementing an existing specialized life sciences, medtech, pharmaceutical, or biotech agentic AI solution mitigates these complexities significantly. Specialized solutions come ready-equipped with agentic workflows, prebuilt reflection patterns, domain-specific embeddings, and optimized integration modules. Pre-tested and penetration-certified, they ensure security and compliance out-of-the-box, with proven consistency in performance and minimized variability.
Immediate Usability and Integration
Prebuilt solutions are typically designed to seamlessly integrate into existing ERP, CRM, and CPQ workflows, significantly reducing implementation friction. Users benefit from predefined schemas, consistent data extraction, and multi-language support, allowing fast deployment and immediate usability without additional manual overhead.
Scalability and Cost Efficiency
At scale, ready-to-use solutions reduce the total cost of ownership through optimized infrastructure, centralized updates, and vendor-provided support. Organizations can immediately benefit from consistent, validated, and standardized analytics without the resource drain of continuous maintenance and internal troubleshooting.
Real-world AI Use Cases from working with the top ten medtech firms
Consider scenarios such as capturing highly detailed award criteria from diverse documentation, accurately extracting nuanced healthcare data, performing country-specific analytics across varying languages, and normalizing the data values and quantities. At a small scale you can resolve some of this with prompt engineering and processing the documents manually. In an enterprise grade solution you may have thousands of opportunities with multi-language scanned documents that need to be processed daily. Such tasks involve highly sophisticated AI orchestrations—tasks that are painstakingly complicated to accurately replicate internally but efficiently addressed by specialized vendor solutions that have already done extensive groundwork and have production-grade core AI components.
Additionally, vendors providing specialized Life Sciences AI solutions have performed extensive penetration testing, satisfying rigorous security standards required for sensitive data handling and regulatory compliance. Attempting such certification independently is both resource-intensive and risk-prone.
Buy vs. Build: Strategic Considerations
To build or to buy? Answering this pivotal question revolves around several key strategic considerations:
- Time-to-Value: DIY solutions are slow to develop, while buying a prebuilt agentic workflow solution provides quicker ROI and value realization.
- Complexity and Risk: Building involves substantial complexity, internal expertise, and continuous risk mitigation, while buying mitigates these significantly.
- Domain Specialization: Existing solutions are already extensively tuned and validated against the nuances of pharma/biotech/medech-specific criteria and regulations.
- Scalability and Maintenance: At large scale, prebuilt solutions offer significantly greater operational efficiency, lowering overhead, and ongoing maintenance burdens.
Conclusion: Thoughtful Decisions, Strategic Impact
While the allure of bespoke AI solutions is understandable, the significant technical, domain-specific, regulatory, and operational complexities associated with building your own solution in Life Sciences cannot be overstated. Specialized AI agentic workflow platforms offer solid integration, robust security and compliance, proven domain specificity, and optimal scalability.
Organizations keen on strategic advantage and swift ROI realization will likely find that choosing to buy an existing specialized Life Sciences AI solution significantly reduces complexity and cost, ensuring robust and immediate operational enhancement. Building your own AI solution, though attractive in theory, presents substantial and nuanced practical hurdles that few organizations are fully prepared to overcome efficiently.
In navigating AI in Life Sciences, recognizing when to leverage existing specialist AI partners such as Vamstar (I’m the CTO and co-founder) can be your greatest strategic advantage, rapid deployment and return on investment.
Other Articles
6 minutes read
Why Net Price Outweighs List Price in Pharma and Biotech Markets
As the pharmaceutical and biotech landscapes evolve, reliance on list prices has become an increasingly outdated approach. List prices provide a static, oversimplified figure that doesn’t capture the market complexities that companies need to navigate. In today’s environment, net price intelligence—the actual price after all discounts, rebates, and negotiations—provides a more accurate, actionable view of the market. This intelligence is essential for strategic growth and sustainable market access.
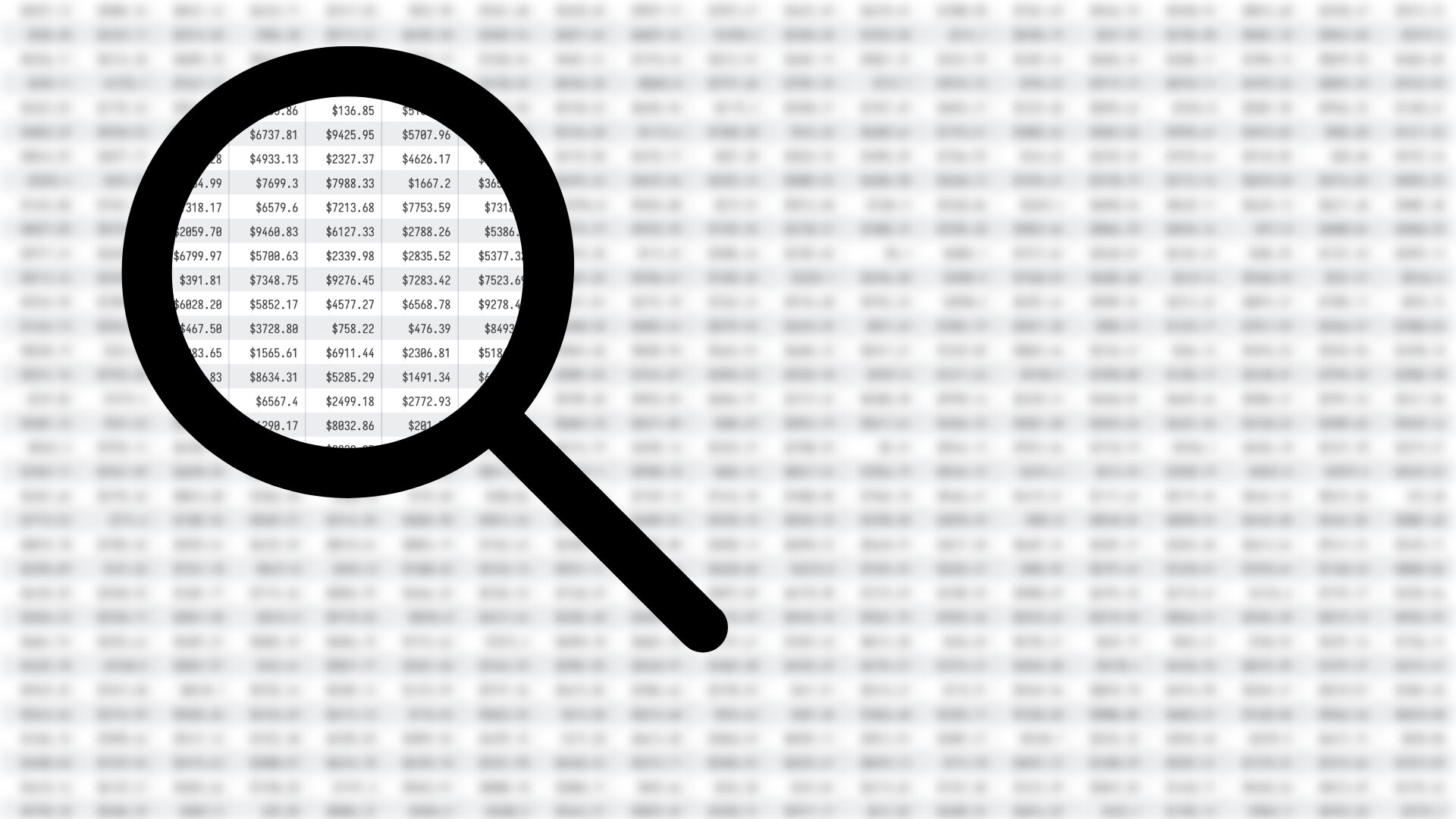
Register to continue reading.
7 minutes read
Navigating the AI Act: The Role of Medical Technology in Shaping European Healthcare
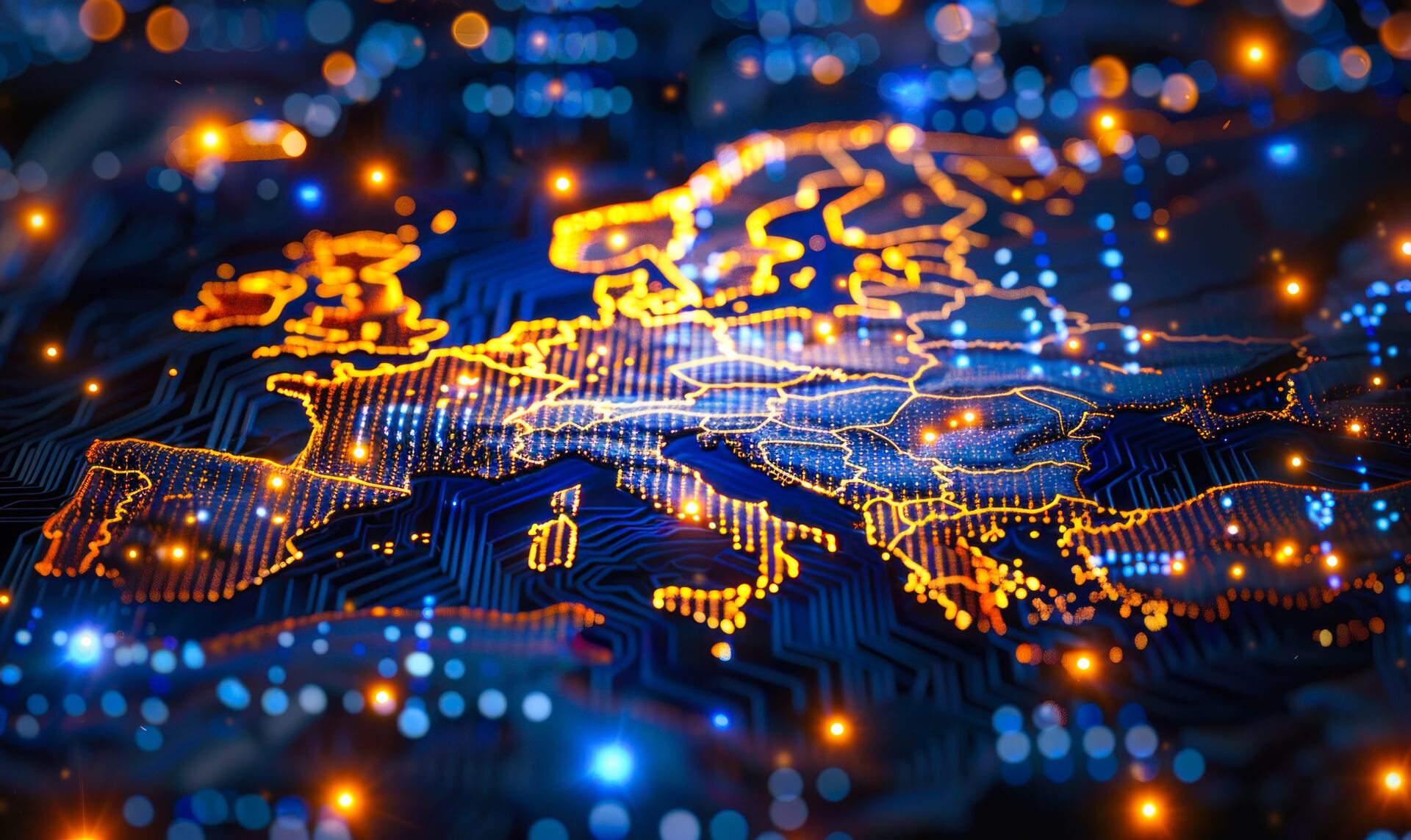
The European Union’s Artificial Intelligence Act (AI Act), which came into force on August 1, 2024, marks a groundbreaking move in regulating the application of AI technologies across various industries, with a particular focus on medical technology. With an emphasis on safety, transparency, and adherence to fundamental rights, the legislation sets the framework for how AI systems are developed and used. For the medical technology sector, the AI Act outlines specific obligations that will significantly influence the design and deployment of AI-enabled devices in healthcare.
Implications for the Medical Technology Sector
The AI Act categorises AI systems by risk levels—unacceptable, high, medium, and low. AI-enabled medical devices, given their potential to directly impact patient outcomes, almost invariably fall under the high-risk category. Consequently, manufacturers of these devices must meet rigorous compliance requirements, including the following critical areas:
- Risk Management: Developing comprehensive frameworks to identify, assess, and mitigate risks related to AI-driven components.
- Data Governance: Ensuring that datasets used to train AI systems are high-quality, relevant, diverse, and free of bias to improve reliability and performance.
- Transparency and Documentation: Documenting the AI system’s design, development lifecycle, and decision-making processes in detail to uphold accountability.
- Human Oversight: Incorporating mechanisms to allow human intervention and override AI-driven decisions, safeguarding the central role of human judgment in critical healthcare scenarios.
- Accuracy and Robustness: Demonstrating the reliability, precision, and adaptability of AI systems across various operating conditions.
Beyond these provisions, compliance with the AI Act integrates with the existing requirements of the Medical Device Regulation (MDR) and the In Vitro Diagnostic Regulation (IVDR), further heightening the regulatory demands placed on medical technology companies.
Industry’s Response and Actions
The medical technology industry, represented by organisations such as MedTech Europe, recognizes the importance of a harmonised regulatory approach and has voiced its support for the EU’s efforts. However, certain challenges remain. MedTech Europe recommends further refinement and clarification to ensure the AI Act not only addresses safety but also encourages innovation and the seamless adoption of AI in healthcare.
Key recommendations include:
- European Commission Guidelines: Advocacy for swift publication of compliance guidelines to provide clear instructions for stakeholders.
- Alignment of Standards: Calls for consistency between the AI Act and existing medical technology standards to eliminate conflicts and redundancy.
- Unified Conformity Assessments: Promotion of consolidated assessment procedures to simplify compliance processes and reduce administrative costs.
Medical device manufacturers are already taking proactive steps to align with the AI Act. Many companies are implementing robust compliance frameworks, which include enhanced quality management systems focusing on risk management, data governance, and human oversight throughout the AI lifecycle. Simultaneously, they are preparing for third-party conformity assessments to meet both AI Act and pre-existing MDR/IVDR obligations.
Challenges and Key Considerations
While the AI Act is a significant milestone, it introduces additional regulatory complexities that could disproportionately impact small and medium-sized enterprises (SMEs) in the medical technology sector. Recognizing this, the legislation includes measures such as priority access to regulatory sandboxes and support from European Digital Innovation Hubs to offer SMEs guidance and resources for compliance.
Another key consideration is the increased burden on notified bodies, which conduct conformity assessments. Meeting the requirements of the AI Act necessitates specialised expertise in AI technologies, leading to recruitment challenges, higher operational costs, and potential delays in the approval process.
Looking Ahead
The AI Act is poised to shape the future of AI-enabled medical technology in Europe, setting ethical boundaries and ensuring safety while fostering innovation. Although the additional compliance requirements create challenges, particularly for SMEs, the medical technology industry is actively engaging with regulatory bodies and adapting its practices to meet these demands.
By implementing effective compliance strategies and collaborating with policymakers, the industry aims to integrate AI innovation into healthcare systems while ensuring that patient safety and care quality remain at the forefront of its efforts. The AI Act signals a new era of regulation—one that balances technological advancements with ethical and safety considerations to drive meaningful improvements in European healthcare.