7 minutes read
Why Conglomerate Operating Systems Are Stifling Commercial Innovation in Life Sciences
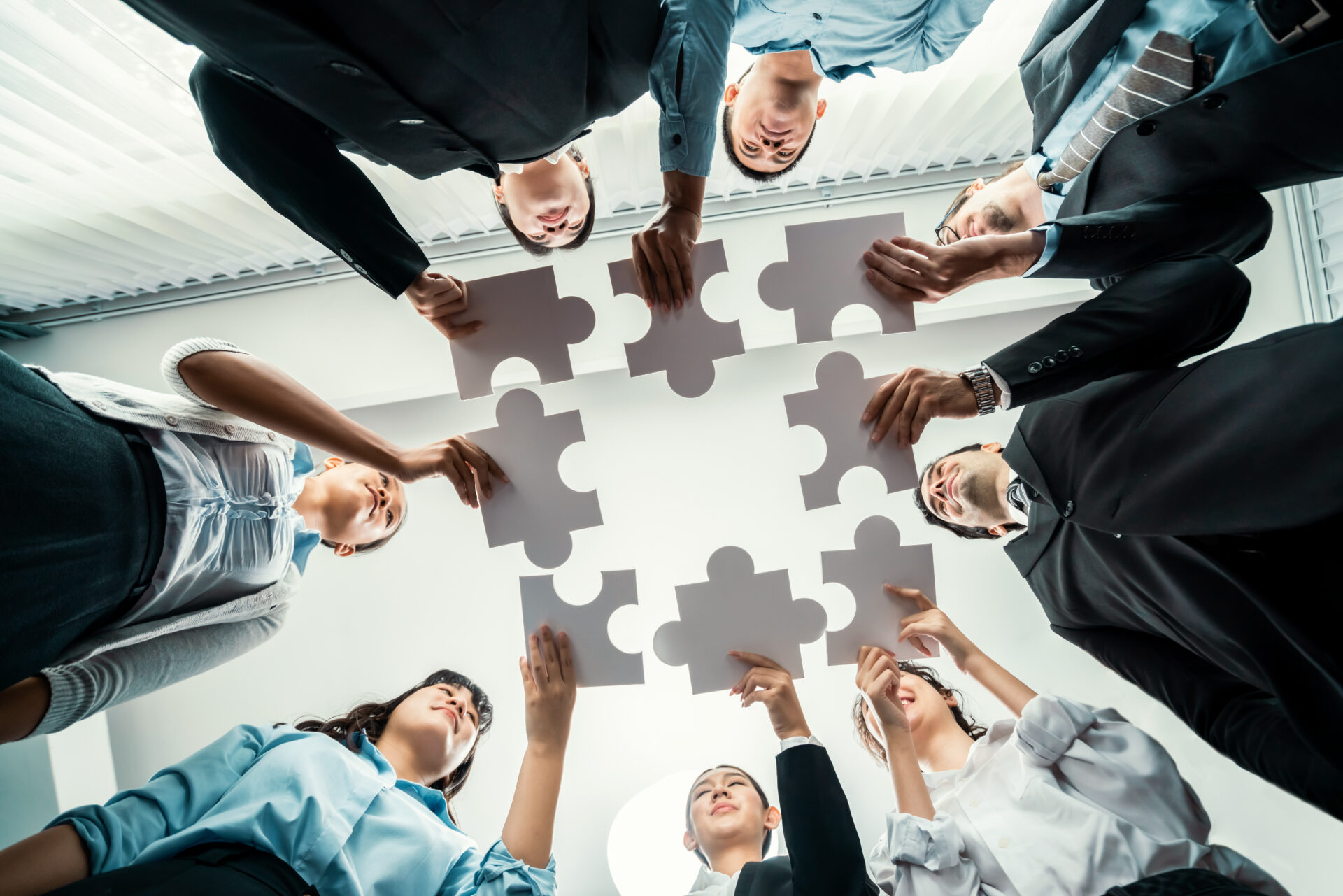
In the life sciences sector, conglomerates like Danaher, Thermo Fisher Scientific, GE HealthCare, and others have excelled at driving internal product innovation and enabling client-side breakthroughs. Their operating models—systems such as the Danaher Business System (DBS) or Thermo Fisher’s Practical Process Improvement (PPI)—have become gold standards for operational excellence. However, these same systems that catalyze product and process innovation are increasingly hampering commercial innovation. Specifically, they are slowing the adoption of AI-driven solutions, modern go-to-market strategies, dynamic pricing models, and customer orchestration capabilities critical for the future of healthcare and life sciences.
The Strength of the Machine: Conglomerate operating systems bring undeniable strengths: relentless focus on continuous improvement, deep-rooted Lean and Six Sigma principles, and operational discipline. These frameworks have been instrumental in:
- Scaling manufacturing precision.
- Improving R&D cycle times.
- Standardizing quality across complex portfolios.
Their impact on product excellence and customer enablement has been transformative, particularly in complex verticals such as bio-manufacturing, precision diagnostics, and MedTech innovation.
Access this article
Please enter your details to continue reading.
Subscribe to continue reading.